Low Fidelity
Low Fidelity
Low Fidelity
Complaint Analysis
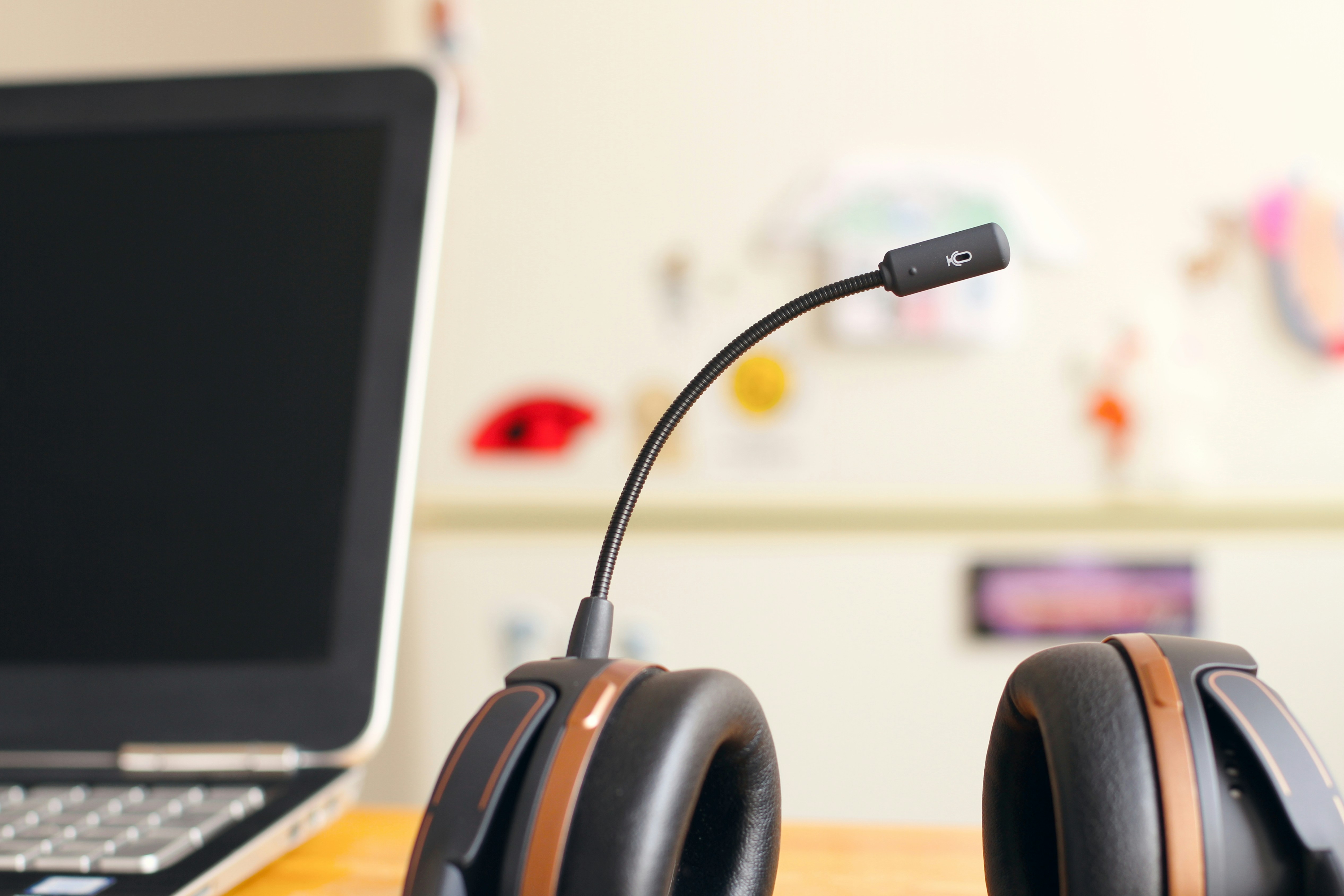
Overview
Complaint Analysis is a data-driven validation experiment that helps us identify the most pressing customer pain points by analysing historical support tickets, complaints, and service inquiries. At Future Foundry, we use this test when we need to uncover recurring friction points, feature requests, and areas where customers struggle most before developing new solutions. Unlike surveys, which rely on self-reported feedback, this experiment taps into real-world frustration data—what customers actively complain about, what confuses them, and where they consistently need help. This makes it particularly valuable for B2B SaaS, consumer tech, and service-heavy industries, where strong customer experience is a key differentiator.
Complaint Analysis is a data-driven validation experiment that helps us identify the most pressing customer pain points by analysing historical support tickets, complaints, and service inquiries. At Future Foundry, we use this test when we need to uncover recurring friction points, feature requests, and areas where customers struggle most before developing new solutions. Unlike surveys, which rely on self-reported feedback, this experiment taps into real-world frustration data—what customers actively complain about, what confuses them, and where they consistently need help. This makes it particularly valuable for B2B SaaS, consumer tech, and service-heavy industries, where strong customer experience is a key differentiator.
Complaint Analysis is a data-driven validation experiment that helps us identify the most pressing customer pain points by analysing historical support tickets, complaints, and service inquiries. At Future Foundry, we use this test when we need to uncover recurring friction points, feature requests, and areas where customers struggle most before developing new solutions. Unlike surveys, which rely on self-reported feedback, this experiment taps into real-world frustration data—what customers actively complain about, what confuses them, and where they consistently need help. This makes it particularly valuable for B2B SaaS, consumer tech, and service-heavy industries, where strong customer experience is a key differentiator.
Process
We work with customer support teams or CRM systems to extract historical support tickets, live chat logs, and complaint records. Once themes emerge, we validate them by cross-referencing complaint data with customer churn, Net Promoter Scores (NPS), and feature adoption rates. If a particular issue is strongly linked to cancellations or negative reviews, solving it could unlock significant value. The final step is to prioritise insights—some pain points may indicate minor usability tweaks, while others may reveal deeply unmet needs that justify new product development.
We work with customer support teams or CRM systems to extract historical support tickets, live chat logs, and complaint records. Once themes emerge, we validate them by cross-referencing complaint data with customer churn, Net Promoter Scores (NPS), and feature adoption rates. If a particular issue is strongly linked to cancellations or negative reviews, solving it could unlock significant value. The final step is to prioritise insights—some pain points may indicate minor usability tweaks, while others may reveal deeply unmet needs that justify new product development.
We work with customer support teams or CRM systems to extract historical support tickets, live chat logs, and complaint records. Once themes emerge, we validate them by cross-referencing complaint data with customer churn, Net Promoter Scores (NPS), and feature adoption rates. If a particular issue is strongly linked to cancellations or negative reviews, solving it could unlock significant value. The final step is to prioritise insights—some pain points may indicate minor usability tweaks, while others may reveal deeply unmet needs that justify new product development.
Requirements
Running this experiment requires access to customer support records, a structured method for categorising feedback, and a way to link customer issues to business impact. The most valuable insights come when we combine support data with qualitative customer interviews to understand the deeper reasons behind frustrations.
Running this experiment requires access to customer support records, a structured method for categorising feedback, and a way to link customer issues to business impact. The most valuable insights come when we combine support data with qualitative customer interviews to understand the deeper reasons behind frustrations.
Running this experiment requires access to customer support records, a structured method for categorising feedback, and a way to link customer issues to business impact. The most valuable insights come when we combine support data with qualitative customer interviews to understand the deeper reasons behind frustrations.
Discover other experiments
Explore more real-world experiments that have helped teams validate ideas, reduce risk, and accelerate innovation.